Generative AI 101 Slides
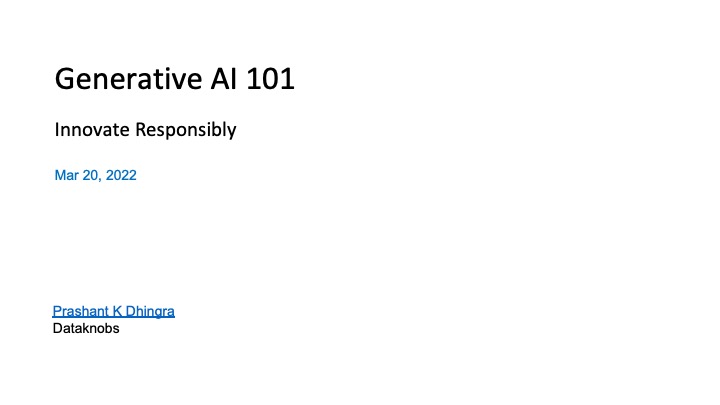
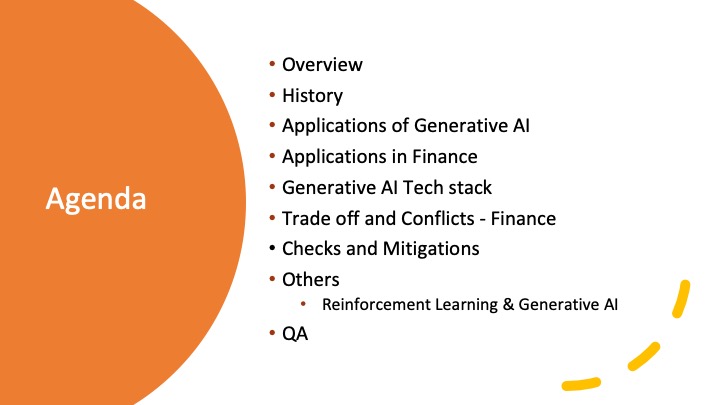
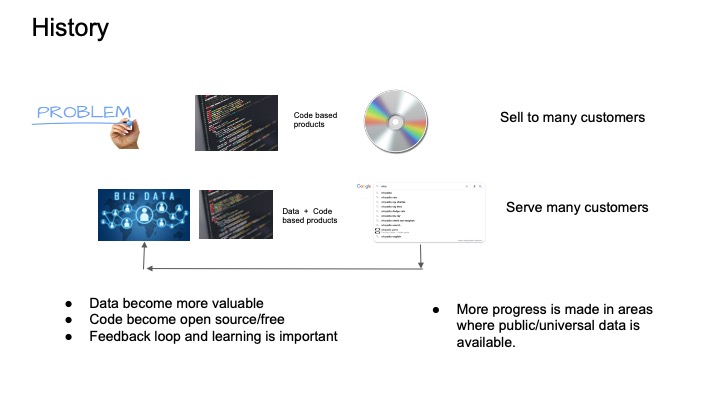
Learn how software evolved from coding to data driven ML. Now genertive AI is even generating data and code. This will change product business model and also product management.
Learn how software evolved from coding to data driven ML. Now genertive AI is even generating data and code. This will change product business model and also product management.
Foundations models are train on broad and generic data. One can use propriety data and use these models for specific tasks in specific domain e.g. finance, health New businesses can be built by training model on top of foundation model. Key is propriety data, domain knowledge and workflow.
Many verticals are impacted because of progress in generative AI. This is just a start and more advance applications will evolve. Applications specific to propriety dataset for doing a narrow task will also evolve. More importantly generative AAI can solve cold start problem by generating dataset for model training.
Finance can benefit from applications that help investors, portfolio managers.